The growth of data generation has given a boost to data science, and while we’re racking up billions of bytes of data on a daily basis, it’s no surprise that data scientists are more needed than ever if we want to make use of all this data.
However, just like data science is on a pretty fast track, AI is not lagging behind, and there’s lots of development going on in that sector as well, with machines growing smarter and autonomous day by day.
This initially sounded like a victory to us humans that machines will help us in most process-hungry jobs; it’s high-time when it started being a threat to most people stealing their bread-earning.
Amongst all other fields, it’s becoming a threat to data science amongst the top tier, and most aspiring data scientists nowadays worry about this. In fact, according to a study done by McKinsey, around 64-69% of the total time spent in the US, collecting and processing data could be automated using AI.
While AI certainly has the potential to perform the tasks usually attributed to a Data Scientist, such as data collection and data analytics, there are various other factors that most of us miss. So, in this article, I’m going to explore everything you need to know about AI replacing Data Science and if it’s even possible or not.
Highly Recommended Articles:
The Future of Business Analytics: Is it Automation?
Will Data Science Die? (And How is Data Science Evolving?)
Without any further ado.
Will AI Replace Data Science?
To understand this, we first need to segregate data science jobs. Data Science is a wide field that has several jobs under its hood, ranging from data analytics to building ML solutions; the two main categories are:
- Data Engineers: They’re the ones who are responsible for the data collection, performing different operations on it such as data cleaning, and finally warehousing that data to be in a format that’s ready to use for further analysis.
- Data Scientists: They are the ones who do the most tricky part. Data scientists use the data prepared by the data engineers to perform exploratory analysis and build models to solve various solutions.
Now there are multiple roles of data engineers that AI is threatening to overtake, but there is no significant danger to the data scientists way into the future. Here’s a short summary of some roles which could be expected to be automated in the future.
- Collecting the data from various sources and combining them.
- Cleaning and delivering the data to the places where it’s needed.
- Deploying the models automatically once they’re ready.
- Detecting certain trends in data.
- Generating variations of certain models.
But don’t get me wrong, even though a subset of data engineers’ major tasks are being automated or are currently automated. There will still be a need for data engineers on the market as not everything can be automated, including some of the core tasks such as:
- Supporting data while keeping it optimized
- Coming up with non-SQL pipelines
- Managing the core infrastructure of data
Also, just as computers did not replace humans and instead ended up being very helpful to them in achieving new heights, the same is the case here.
So, everyone out there worrying about AI replacing them in their jobs, sit back, and enjoy AI’s development into becoming a tool for helping you do your job even better.
Why Can’t AI Take Over Data Scientists Jobs?
So, what exactly is the reason we’re putting data scientists in the safe zone? What do they have which cannot be automated and possibly done by the machines keeping in view how smart they are becoming day by day?
Even the most complex games such as Dota 2, which requires quick reactions and strategies have nowadays been dominated by some high-level AI’s, so where do they exactly lack that they cannot possibly do what data scientists can? You’ll get all your answers in this section.
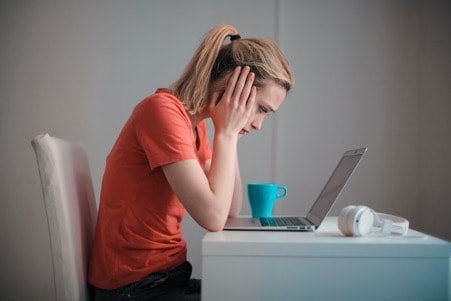
1. Data Scientists Keep Facing New Scenarios
Unlike data engineers’ job, who often come across the same set of tasks such as gathering the data from a given source or cleaning it, data scientists’ job is very versatile. They keep facing new tasks in different scenarios, and they hardly come across the same thing more than a couple of times.
Sadly, this isn’t something AI is great at. Artificial intelligence needs to be trained on a particular job, and only after achieving a certain level of accuracy it can be deployed for that job.
Figuring out new complexities isn’t the strong-suite of an AI, no matter how good it is.
For example, a data scientist’s job includes looking at some business problem and the data available along with it. According to that specific problem domain, a data scientist will develop a specific model that could aim to solve a particular problem. However, this isn’t the main problem.
The major problem is that, most likely, a data scientist may be facing this particular scenario for the first time ever. Still, with his/her expertise and grip on machine learning models and their development, he’ll be able to entertain the task one way or the other.
In contrast, if the process were automated, an AI system would be buried under problems, and it’s improbable it would solve a problem it’s never faced before. Moreover, you certainly cannot train an AI on every possible problem we could ever face.
This is the most primary difference between the two. Data scientists are highly adaptable, while AI, not so much. It’s only good where you keep facing similar situations. Or if you don’t, at least you have a limited domain for which you can program your AI. But when the domain is limitless, it’s certainly beyond the scope of AI.
2. Machines Don’t Have Intuition
No matter how smart an AI system becomes, it’s still a computer at the end of the day. You can’t compare the smartest AI on the planet with the person having the lowest IQ. Machines simply don’t have the brain we do. They rely on data and how they’ve been trained, and that’s pretty much the end to it.
However, most of the time, when we’re working commercially, there are scenarios where a bit of Intuition is needed. Data doesn’t always speak the truth, and a bit of common sense always comes in handy.
Nevertheless, some recent progress has made the computers come as close to common sense as possible, but it still needs some work, and I don’t see it happening for a considerable amount of time. Let’s see an example to make things extra clear in your mind.
A company that goes by the name “Elemental Cognition” conducted an experiment on their advanced language program powered by their state-of-the-art AI. They left the sentence unfinished at “Zoey moves her plant to a sunny window. Soon …” and the rest was left to AI to fill.
Surprisingly, well, not so surprising for me, the AI failed to complete the sentence.
On the other hand, a human would have completed it by merely appending “the sun will help the plant grow healthy” or something this sort at the end since it’s a pretty common thing that plants need sunlight to stay healthy.
So, this is what I’m emphasizing here.
While AI can certainly perform great and even beat humans when it comes to something they could train upon, they can never compete against a human brain when it comes to general observations or common sense.
Highly Recommended Article: 7 Key Differences between Machine Learning and AI
This is a considerable gap, and while this gap remains between the human being and AI systems, we will never be able to automate jobs that require critical thinking and Intuition, i.e., Data Scientist.
3. AI Doesn’t Have the Soft Skills
Suppose we suppose for a moment that AI has reached up a point where it has all the technical skills equivalent to a data scientist, and hence data science is fully ready to be automated.
The question is: are technical skills all that’s needed to become a data scientist? Let’s see.
Even if AI manages to have all the technical skills it needs to develop models and any wrangling of the data as per need, can it effectively explain how their model will make the lives of the customer better?
Can they convey what effect their new algorithm has on the existing scenario or the customer’s lives?
Do they have the required soft skills to do so?
Well, the simple and realistic answer to that is “No.” As good as AI could get, it could never compete with a data scientist’s interpersonal skills or even those of a regular executive in a company.
Surprisingly, according to LinkedIn, 92% of the HR professionals look for soft skills in candidates and think they matter at least as much as the technical skills, if not more.
Lastly, people also find it hard to trust AI – which comes with their reasons. Once, an algorithm was used to sentence criminals, and when later studied, it was found to be discriminating prisoners based on their races. It was later banned, but obviously, it won’t be soon when people start trusting AI completely.
Why AI Might Be Good for Data Scientist
If we try to visualize the situation optimistically, AI might as well prove to be an excellent asset for data scientists rather than being a threat. Let’s see some of the top reasons why AI will complement and not replicate the data scientists.
- They Can Save Their Time from The Boring Tasks
There are various tasks in the pipeline of ML models that data scientists have to go through, whether they like it or not. One such process is ETL; I’m sure if you have also gone through the process, it feels pretty boring since every time you need to go through the same steps.
Such steps can be easily automated using AI, and as a result, data scientists can save a lot of their time and spend it where it really matters.
- AI Can Help Identifying Tiny Patterns
AI is great when it comes to consistent but hefty work. It could leave even the masters of data science behind when it comes to studying long raw forms of data and looking for the slightest inconsistencies. Moreover, if you’re familiar with visualizing data and looking for small patterns, you probably know how energy-draining of a task it is. Luckily, this can also be automated and save a lot of your energy.
- Humans Cannot Beat AI in Accuracy
As we discussed previously, there are a variety of tasks within the pipeline of building a model that can be automated.
Once they’re successfully implemented, AI will swift through the process, with their efficiency being unmatched to that of a data scientist, no matter how highly qualified or experienced.
Some processes are fully automated to date, such as modeling. These processes are done in an extremely fast manner, and if not for AI, data scientists would find it very hard to be as efficient as they are today.
Final Words
Remember our Initial Question: WILL AI REPLACE DATA SCIENCE?
The world of Artificial Intelligence is advancing quickly, and we’re seeing a massive paradigm shift when it comes to conventional workflows.
Along with its development, it’s threatening many jobs, and human presence is diminishing, with automation being the new thing.
Amongst all these professions, people are afraid it might hit data science with the massive momentum it’s proceeding with. However, throughout the article, we saw how it’s too soon to say if data science can be automated. There are various gaps yet to be fulfilled by AI, which doesn’t seem to come by anytime soon.
We discussed how AI could be an extremely useful asset to a data scientist rather than being a threat on the upside. It can save data scientists from a lot of tedious and repetitive processes and let them focus where it matters.
This way, AI might actually be a blessing in disguise when it comes to data science. While it may capture some of the data engineering markets, it’s certainly not a threat to data scientists, at least for the foreseeable future.