Sampling is an inherent human trait we follow whenever we want to study something, but the domain is huge enough to force us to base our study on a sub-sample only. This is true for most of the studies in the practical world. The whole population is never accessible; even if it is, it’s not worth going through all of it.
Sampling lets us cleverly study the characteristics of a vast population without actually going through it all. Not only does this make the studies quicker and more practical, but it also helps reduce costs considerably. So, today in this article, we will see in-depth all the different techniques one can employ to do sampling. Moreover, we’ll also see how you can choose the best sampling technique depending on your scenario. So, let’s jump in!
Why Do We Need Sampling Techniques?
Research benefits greatly from sampling. It is one of the most crucial elements that affect how accurate your study or survey results are. If your sample contains any errors, the outcome will be affected accordingly. Depending on the situation and necessity, numerous methodologies aid in sample collection. But before diving into the topic, let’s look at some essential statistical terms you might need to remember.
- Population:
A population is a group of related objects or occurrences relevant to a particular topic or experiment.
- Sample:
It is the particular group from whom you will get data. The sample size is always smaller than the population as a whole.
- Sample unit:
It is the object or person being observed.
- Sampling frame:
It is a list of everything in the population that can be observed, whether it be people or other objects.

What is a Sampling Technique?
It is seldom possible to gather data from every member of a group of individuals when conducting research on them. So, what do you do? Well, you pick a sample instead. The population that will actually take part in the study is the sample.
The sampling technique is the method you employ while choosing a sample from a population. For example, you could select every 3rd person, everyone in a particular age group, and so on. You must carefully consider your study before choosing an appropriate sampling technique. It has a significant effect on your results. For example, some sampling techniques might be intentionally biased. So, selecting a suitable sampling technique is essential to draw accurate conclusions from your data.
What are the different types of Sampling Techniques?
There are two significant types of sampling techniques which are then divided into sub-types:
1. Probability Sampling
2. Non-Probability Sampling Techniques
Let’s go through both, along with their sub-types.
Probability Sampling Techniques
Using a set of predetermined criteria and a random selection of population members, a researcher uses the sampling technique known as probability sampling. With this selection criteria, each member has an equal chance of being included in the sample. Our best shot at producing a sample that is accurately representative of the population and enables us to draw robust statistical conclusions about the entire group is through probability sampling. Random sampling is another name for it. It has four sub-divisions:
1. Simple Random Sampling Technique:
Every person in the population has an equal probability of getting chosen in a simple random sampling. The entire population should be part of your sampling frame. The Simple Random Sampling method is one of the top probability sampling approaches that aid in time and resource conservation. It is a reliable way to gather information.
The fact that this method is the most straightforward for probability sampling is a significant benefit. It does, however, come with a disclaimer: it might not choose enough people who fit our criteria. We use it when we don’t know anything about the target population beforehand.
Example:
A company has decided to give a bonus to 10 of its employees. These employees will be selected randomly through any method from the whole company.
2. Systematic Sampling Technique:
In systematic sampling, the first person is chosen randomly, and the others are selected according to a predetermined sampling interval. Put each person, in the population, in some kind of order and select every nth member to be in the sample from a random starting point.
This approach is easy to use, especially with large populations. A possible disadvantage could be if there is an underlying pattern in how we are choosing objects from the population, it could potentially result in bias.
Example:
Suppose you need to choose a sample of 50 people from a population of 100. You will select every 2nd person on the list.
3. Stratified Sampling Technique:
Stratified sampling entails breaking the population up into smaller groups that might have significant differences. Ensuring that each subgroup is fairly represented in the sample enables you to reach more accurate findings. You can employ this sampling technique by dividing the population into smaller groups or strata according to the pertinent property (e.g., gender, age range, residence area, etc.). You determine the appropriate number of individuals to sample from each subgroup based on the population’s overall proportions. Then you choose a sample from each subgroup using random or systematic sampling.
We employ this sort of sampling when seeking representation from all the population’s subgroups. However, stratified sampling necessitates thorough familiarity with demographic characteristics.
Example:
A researcher wants to know the number of people in a country who went to college. He\she would divide the country into cities and then further divide the cities into age groups. He\she would then randomly select a sample to get information about the topic.
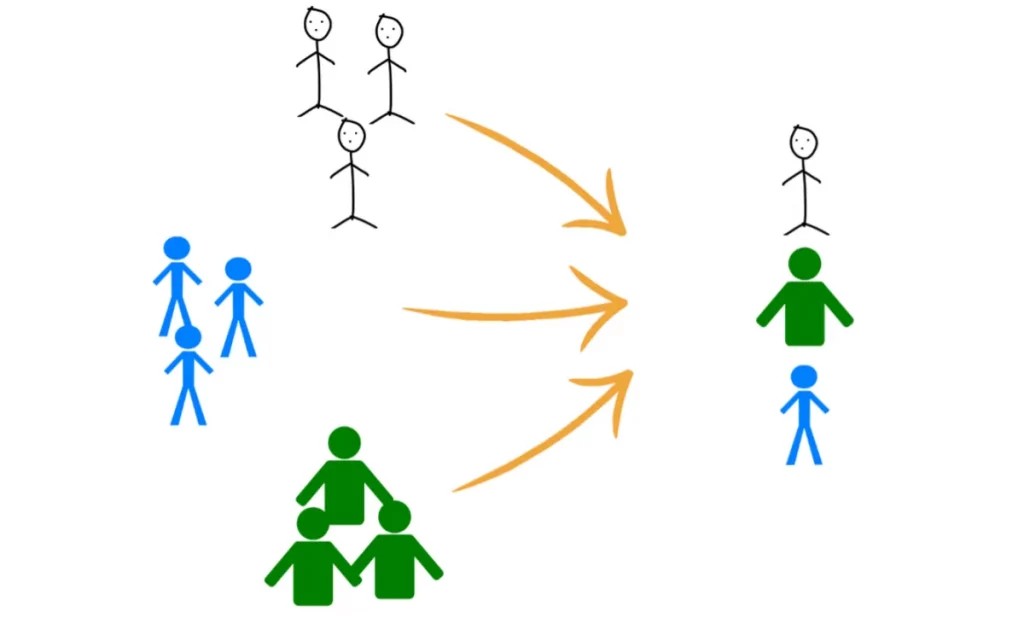
4. Cluster Sampling Technique:
In cluster sampling, we break down the overall population into smaller groups, each of which shares the features of the population as a whole. We also choose the entire subgroups randomly rather than merely picking people. You might incorporate each person from each sampled group if it is practically feasible. If the clusters are large, you can also sample people from each cluster using one of the methods mentioned above.
The sample has a higher chance of mistakes because there may be significant differences between clusters, but it is pretty helpful for handling oversized and dispersed populations. It is challenging to ensure that the sampled clusters accurately reflect the entire population.
Example:
A mobile company is looking to survey people from a country about the usage of phones. It would divide the country into cities, known as clusters, and then further divide the cities into areas (clusters) that are more populated.
Non-probability Sampling Techniques
In non-probability sampling, participants are chosen at random by the researcher. This type of sampling is not a set or predetermined selection procedure. Due to this, it is challenging to ensure that every component of a population has an equal chance of being represented in a sample. It enables simple data collection. A non-representative sample that cannot yield generalizable conclusions carries considerable risk. Non-random sampling is another name for this.
1. Convenience Sampling Technique:
Because participants are chosen based on their availability and willingness to participate, this sampling technique may be the simplest. Only those people who are easily accessible and available to participate in the study are included in convenience sampling.
Although it is quick and affordable, this method cannot yield generalizable conclusions because it is impossible to determine whether the sample reflects the population. Considering how simple it was for the researcher to conduct the study and contact the subjects, it is frequently referred to as convenience sampling. Researchers with almost no authority choose the sample components, and they are selected entirely based on accessibility rather than representativeness.
When gathering feedback is time and money-constrained, this non-probability sampling technique is used.
Example:
For example, if a person is conducting a study about the use of shampoo, they would go to the people they know instead of the general public.
2. Purposive Sampling Technique:
In the purposive sampling technique, the researcher uses their knowledge to choose a sample that will be most helpful to the research’s objectives. This sort of sampling is also known as selective or judgment sampling. It is frequently employed when the researcher prefers to learn in-depth information on a particular occurrence versus drawing general conclusions from statistics or when the population is relatively tiny and focused.
Example:
For example, if a researcher wants to gather information about a particular religion, they should go to the area where it is practiced the most.
3. Snowball Sampling Technique:
When subjects are challenging to trace, researchers use the snowball sampling technique. To discover people who are interested in participating in the study, the researcher contacts other people they know. Using the snowball theory, researchers can follow a few categories to interview and gather data in situations where it is challenging to survey people on a particular topic. This sampling strategy is also used by researchers when the subject is highly delicate and taboo. The population expands like a snowball as a result of this referral strategy. This sampling technique works well when it’s challenging to pinpoint a sampling frame.
Snowball sampling carries a considerable risk of selection bias because the people who are referred will have characteristics in common with the person who refers them.
Example:
For example, if a researcher is conducting a study about the psychological effects of STDs, the snowball sampling technique would be useful as STDs are considered taboo in most areas.
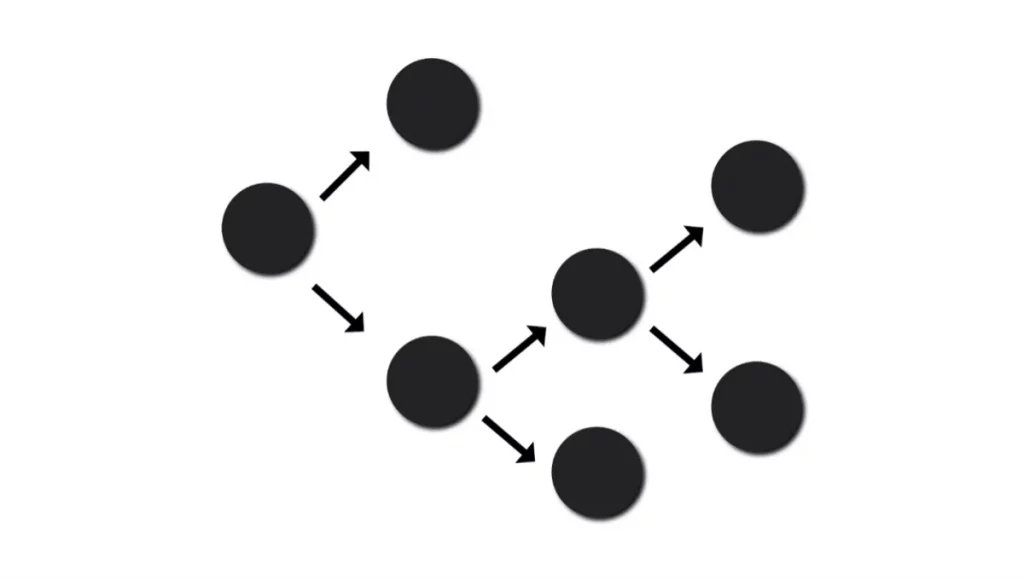
4. Quota Sampling Technique:
The quota sampling technique is conducted based on a predetermined criterion. From the entire population, a representative sample is taken.
This approach divides the sample into groups based on traits and then interviews. The sample should reflect the population regarding the proportion of traits and attributes. The researcher stops collecting data once each group has adequate sample units. This sampling technique has numerous benefits, including its ability to compare groups within the population, quick and uncomplicated execution, and lack of need for a sample frame. The division of the groups may not be correct, and there is a possibility of some bias.
Example:
For example, if our population is composed of 50% women and 50% men, our sample should be composed of the same proportion of males and females.
Conclusion
Sampling is a very extensive yet one of the most undermined areas in research and general statistical studies. People don’t realize how important it is to choose suitable sampling methods to achieve the correct results. Most use the same one or two generic approaches, regardless of their use case, resulting in improper results.
So, to achieve your research objectives properly, selecting a sampling technique carefully while taking everything into consideration is crucial. In order to help you make your decision on a broader category, I’ve made up a table to help you choose the right approach for you, highlighting the major differences between probability vs. non-probability sampling techniques.
Probability Sampling Technique | Non-probability Sampling Technique |
Using a process based on probability theory, samples from a bigger population are picked in the sampling approach known as probability sampling. | Non-probability sampling is a sampling technique in which the researcher selects samples based on the researcher’s subjective judgment rather than random selection. |
The population is randomly selected. | The population is arbitrarily selected. |
The population’s demographics are clearly represented because a procedure for selecting the sample was used. | The portrayal of population demographics is typically biased since the sampling methodology is arbitrary. |
It takes longer to complete since the research design defines the selection criteria before the study starts. | Since neither the sample nor its selection criteria are ambiguous, this kind of sampling technique is quick. |
The research is conclusive. | The research is exploratory. |
Because of the complete neutrality of this form of sampling, the findings are both conclusive and unbiased. | The research is speculative because this highly skewed sampling method affects the outcomes. |