Data cleansing has played an important role in the evolution of data management and analytics. It continues to evolve at a fast pace. Data cleansing is the act of going through all of the data in a system and removing or updating all material that is incomplete, wrong, wrongly structured, duplicated, or unnecessary. Data cleansing typically entails cleaning up data that has been gathered in one location.
Organizations who wish to succeed in their markets must understand the importance of data cleaning in analytics. Data cleaning plays an important role in streamlining many data sources and leads us to improved decision making abilities. Clean data helps in having reliable statistics for a business, thus improves employee productivity and customer engagements.
According to Jack Ma, co-founder and chief executive of Alibaba Group, projections for the future job market, skills associated with data, and analysis will become extremely valuable. Ma says,
“The world is going to be data; I think this is just the beginning of the data period.”
What is Data Cleaning?
The method of detecting and correcting corrupt or defective information from a record collection, table, or database is known as data cleaning, and it entails recognizing missing, wrong, inaccurate, or unnecessary sections of the data along with adding, updating, or removing the messy or coarse details.
Why should we care about data cleaning?
Combining data from various databases can be difficult, and data scientists must check whether the results make sense. The most complicated issues are data shortage and formatting discrepancies. This is what data cleaning is all for. Since real-life data is obsolete, it becomes necessary, emphasizing the importance of data quality control in the industry. 60% of data scientists’ time is spent preparing and cleaning data!
Data cleaning guarantees that you only have the most recent records and relevant files, making it easy to locate them whenever you need them. It also ensures that you don’t have many sensitive details on your computer, which may cause a security risk.
Here’s the importance of data cleansing in analytics:
For businesses that rely on data to keep their projects functioning, data analytics is essential. For instance, companies must determine that valid invoices are sent to the appropriate people. Businesses must prioritize data accuracy to make the most of user data and increase brand awareness. Bill Gates quoted,
“Analytical software enables you to shift human resources from rote data collection to value-added customer service and support where the human touch makes a profound difference.”
Below are some points to shed light on the importance of data cleaning:
Avoid expensive faults
The single best approach is data cleansing for avoiding the costs that arise when organizations are busy handling bugs, correcting erroneous data, or configuring it.
Increase the number of customers
Organizations that keep their records in good condition will create prospect lists based on reliable and up-to-date information. As a result, they improve productivity, increase the amount of consumer acquisition and lower its cost.
Everyone benefits from having reliable statistics. It’s important to provide accurate employee data. It’s beneficial to provide reliable consumer records so you can learn more about your customers and contact them if necessary. You’ll get the best out of your marketing campaigns if you have the most updated and reliable details.
Make sense of data from various sources
Data cleaning leads us down the way for streamlined multichannel consumer data management, enabling businesses to discover innovative ways to meet their target markets and run profitable marketing campaigns.
Enhance overall decision-making abilities
Clean data is the best way to support a decision-making phase. Accurate data supports business intelligence which provides businesses with tools for improved decision-making and operations.
Boost employee performance
Employees who use the data in a wide variety of ways, from consumer retention to resource preparation, get more productive when the databases are clean and maintained. Businesses that actively enhance the quality and precision of their data improve their response time and sales.
Better emailing systems
In the past, incomplete information has been responsible for firms and consumers receiving mail from organizations that are irrelevant to them. This is valid not only for postal mail but also for emails. The more meaningless mail a company receives, the more difficult it is to distinguish critical mail. Data cleansing will minimize the likelihood of businesses receiving irrelevant mail while still ensuring that important messages are not lost along the way and are received and read.
Highly Recommended Articles to Check Next
-
Causal vs Evidential Decision-making (How to Make Businesses More Effective)
In today’s fast-paced business landscape, it is crucial to make informed decisions to stay in the competition which makes it important to understand the concept of the different characteristics and
-
Bootstrapping vs. Boosting
Over the past decade, the field of machine learning has witnessed remarkable advancements in predictive techniques and ensemble learning methods. Ensemble techniques are very popular in machine learning as they
-
Boosting Algorithms vs. Random Forests Explained
Imagine yourself in the position of a marketing analyst for an e-commerce site who has to make a model that will predict if a customer purchases in the next month
Data Cleansing Tips And Methods:
You may be curious how to begin the data cleansing process to understand what it is and why it is so necessary. There is no such thing as a one-size-fits-all solution when it comes to data cleaning. The type of data you have will also determine your data cleaning methods. Here are some general guidelines to get you started.
Deal with missing data
It’s a big risk to ignore missing variables in a data set, and most formulas are unable to recognize them. Any business solves this issue by attributing missing values from other findings or discarding observations of missing values entirely. However, these techniques sometimes result in the loss of data. Companies may mark categorical variables as “Missing” if it is lacking. Missing integer value should be marked and filled in with 0 so that the algorithm can approximate the best constant.
Systemic errors
Systemic errors occur during calculation, file transfer, and other problems resulting from inadequate data management. The most prevalent issues are incorrect punctuation, typos, and incorrectly labeled grades. Such failures effectively demonstrate the significance of data cleaning.
Unnecessary points
Data science organizations often discover unwanted observations in their data sets. These points may be duplicates or ones that don’t apply to the issue they’re trying to solve. Checking for unnecessary results is a smart way to speed up the technical feature creation process – the development team would have a happier time creating prototypes.
How data cleaning helps us?
Businesses and even people also have difficulty cleaning up their data because they keep them for far too long. Data will easily become a jumble, full of statistical and spelling mistakes, redundant data, and confounding, that you won’t be able to find out how it got there in the first place!
The data management process can be greatly improved by data cleaning. Data cleaning is the process for systems, architectures, activities, and procedures to correctly handle an organization’s records. The term “data cleaning” covers a broad range of subjects and helps in many ways.
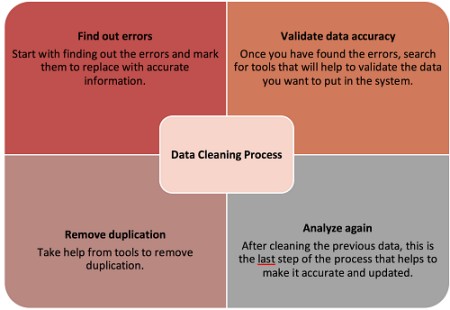
What kind of problems can arise during data cleaning?
The process of data cleaning is necessary and complex at the same time. It often comes with some pitfalls. Some of them are given below.
Typing errors
Typing errors are the most common cause of misspellings. For common words and grammatical errors, incorrect spelling may be found and corrected; but, since databases have a large volume of specific data, it is challenging to identify spelling mistakes at the input stage. Furthermore, it is often difficult to find and fix spelling errors in details such as contact details.
Domain Errors
Domain format errors arise when a value for a particular component is valid but does not adhere to the domain format. For example, a specific NAME database contains a comma to distinguish the first and last names, but the input does not have a comma. Although the input is entirely right, it does not adhere to the domain format.
Confusing errors
When the same actual object is represented by two distinct data values, a confusion mistake appears. For example, in a personal archive, there are two files for the same person with two separate dates of birth. All the other values and identities are the same.
False References
Errors resulting from incorrect results obstruct data validation and trigger data mismatch. For, e.g., if a person enters the wrong reference system value in the department area. Mismatch occurs as a consequence of the data verification process.
Can businesses outsource data cleaning?
As a company’s number of operations grows, it can be challenging to maintain its databases in good condition. And, particularly in challenging areas like machine learning, cleaning data is crucial in developing high-quality algorithms. Only data that has been correctly cleansed will yield a useful market approach and decisions.
It’s a good idea to outsource data set cleaning and maintenance. Businesses may take advantage of additional expertise in a low-cost, low-risk manner without hiring new data scientists.
Outsourcing data cleaning is a scalable approach since the tools are accessible where businesses need them. They can even try out new ideas without having to spend a lot of money upfront.
Concluding thoughts:
Businesses who look after their databases well are awarded these and many other advantages. Organizations that maintain high-quality industry-sensitive expertise achieve a major competitive edge in their markets by rapidly adapting their processes to changing conditions.
Clean data is the foundation for any effective data science project, particularly when developing sophisticated solutions such as machine learning algorithms. Businesses should always take time to clean data and ensure that their projects support the customers and maintain their data collection practices to the fullest extent possible.